Headspace
UI/UX Music Player Concept Design
The Headspace mobile app is an ideal solution for the next step of growth for BPM music player apps in the current market. Because I had yet to come across an app designed to connect the user's current mood to music through any other means than a playlist, it was apparent that there is a need to create a more immediately interactive method of musical beats syncing with heartbeats. With that in mind, I set out to conceptualize an app to facilitate a true mood creating music player.
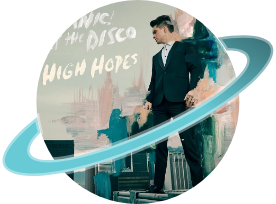
ROLE
Individual Project
4 week
Individual Project
4 week
TOOLS
2D: Adobe XD, Adobe Illustrator, Adobe Photoshop, Adobe InDesign, Adobe After Effects, Adobe Premiere Pro
2D: Adobe XD, Adobe Illustrator, Adobe Photoshop, Adobe InDesign, Adobe After Effects, Adobe Premiere Pro
Context
Despite repeated filtering of playlists, I would always encounter a song that ruins the mood I was hoping for. It is common for people to have a convenient workout playlist to elevate the heartrate, but it is much more difficult to finetune playlists to bring a low mood up or vice versa. In an attempt to see if friends or family had better playlists, we discovered how similar music could result in completely different moods. Though most apps talk about the importance of using the music beat to affect moods, further research shows familiarity with the songs itself could sometimes be more important.
My user research shows that among Americans who stream music, half self-curate playlists, while 35% use public playlists based on genres or moods. Around 38% view playlists as an important part of their streaming experience, and 44% love to create their own playlists. (x)
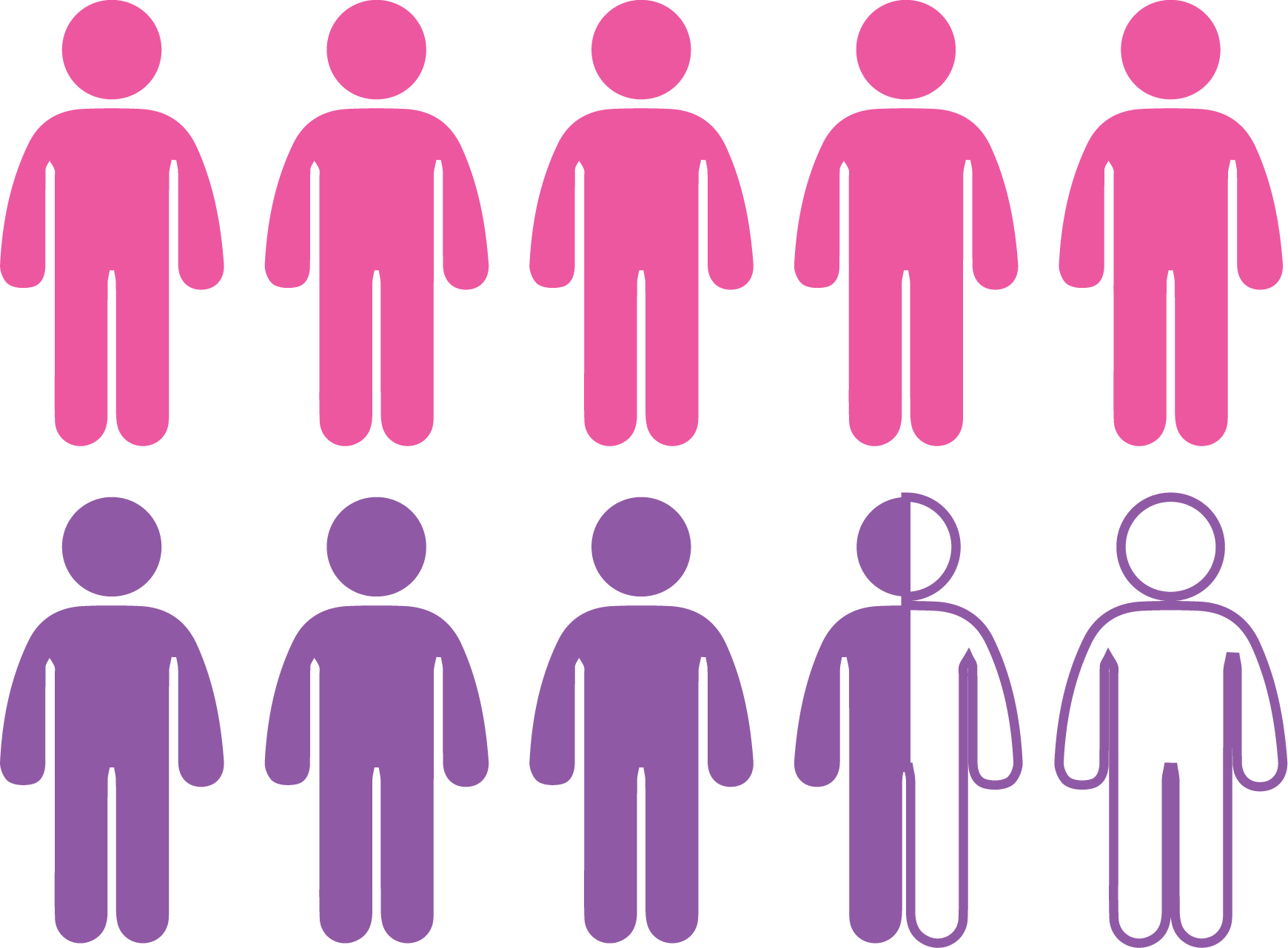
Problem
The tempo and familiarity of songs in playlists are not correlating with the desired mood.
Many people listen to music to help put themselves in a better mood or to help focus on doing a task. Even with a custom playlist, sometimes a song does not match the desired effect of the listener on a given day, interrupting the mood as they try to find the ideal song.
Goals
1. Create an app that tracks HRV (heart rate variability), BPM of a user, and familiar songs to create a playlist that can transition a user's current mood to their desired mood.
2. Track mood history because regulation is a form of prevention.
3. Show past playlists to track change and growth in mindsets.
Research Key Points
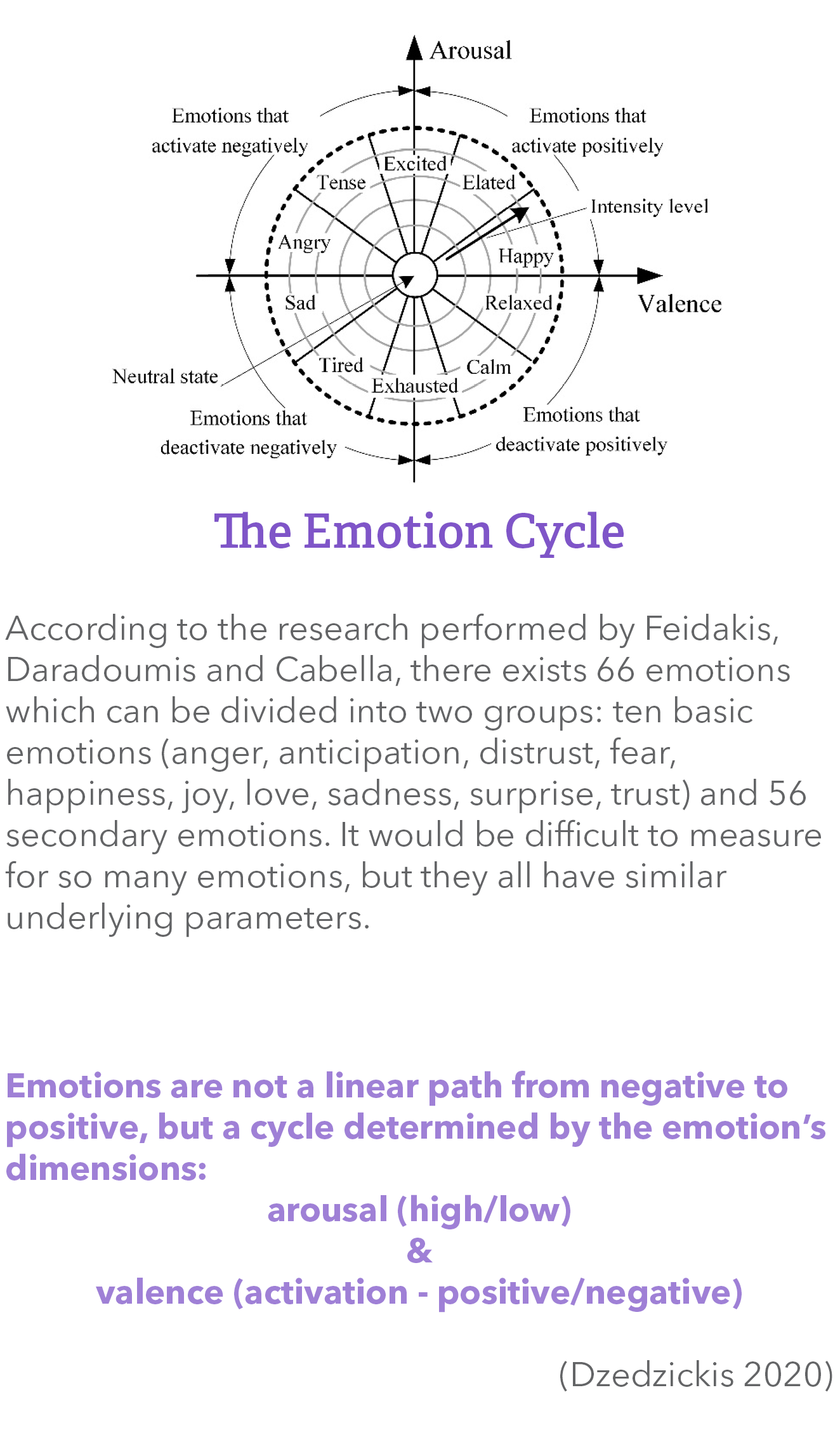
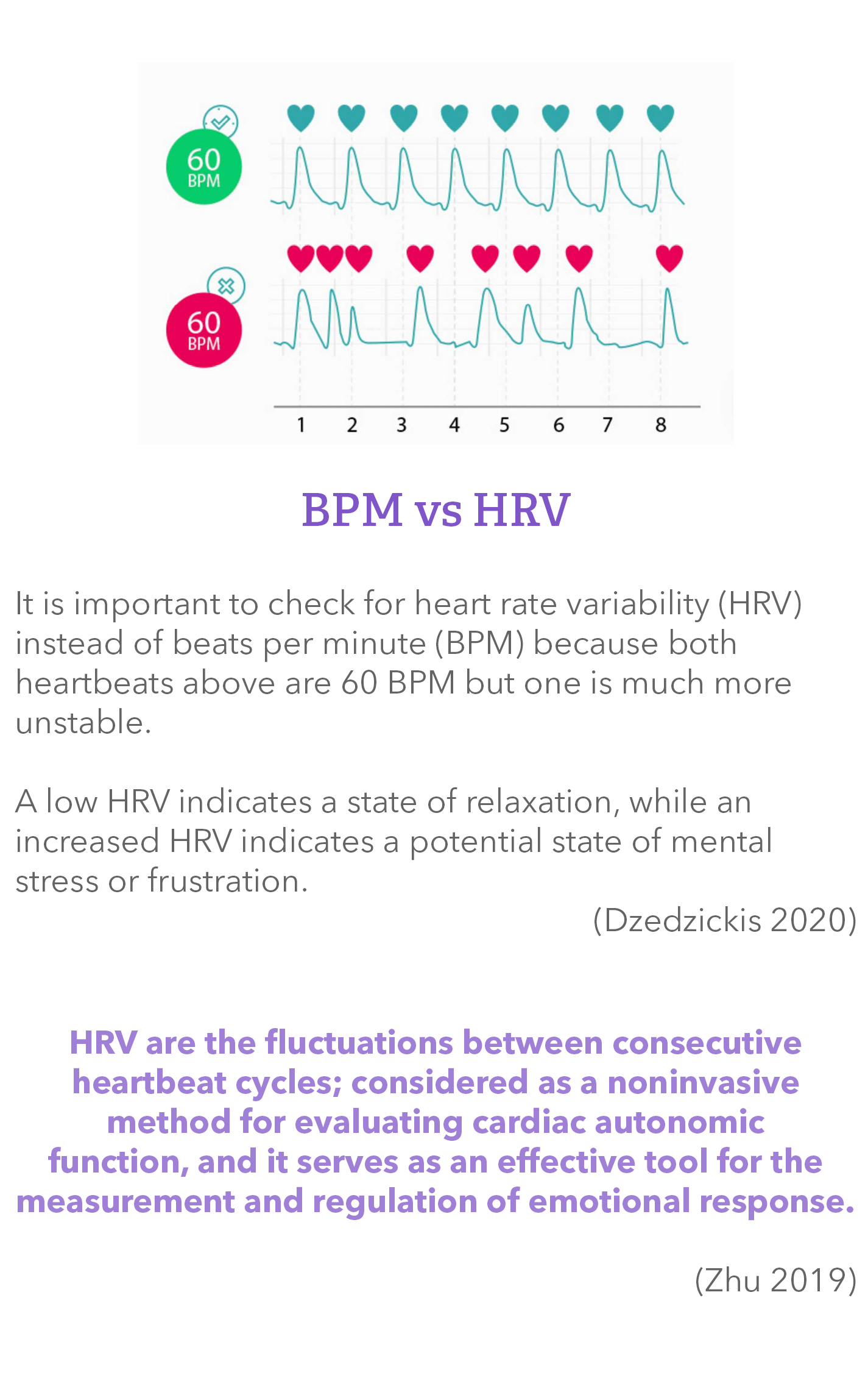
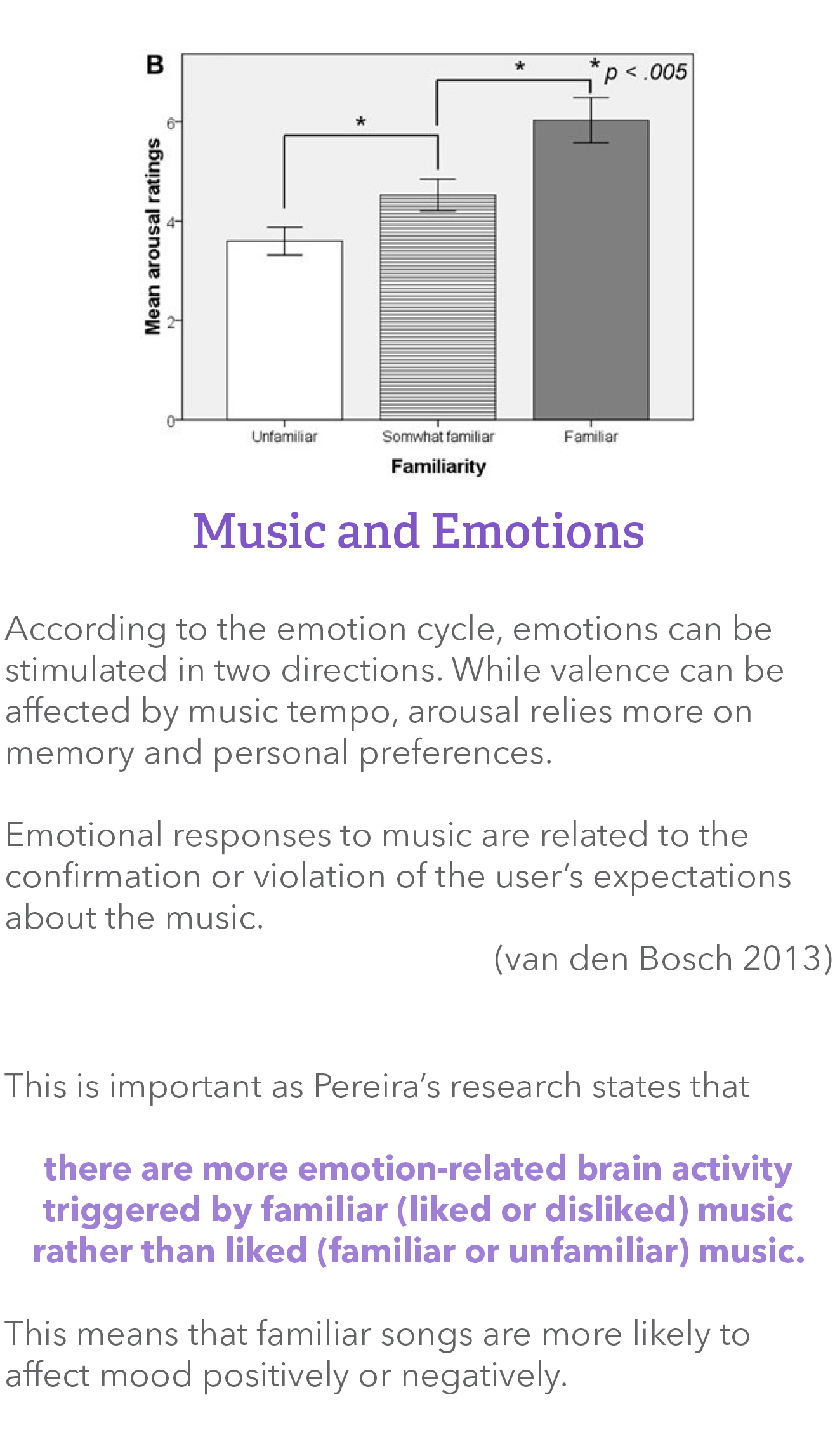
Competitive Analysis
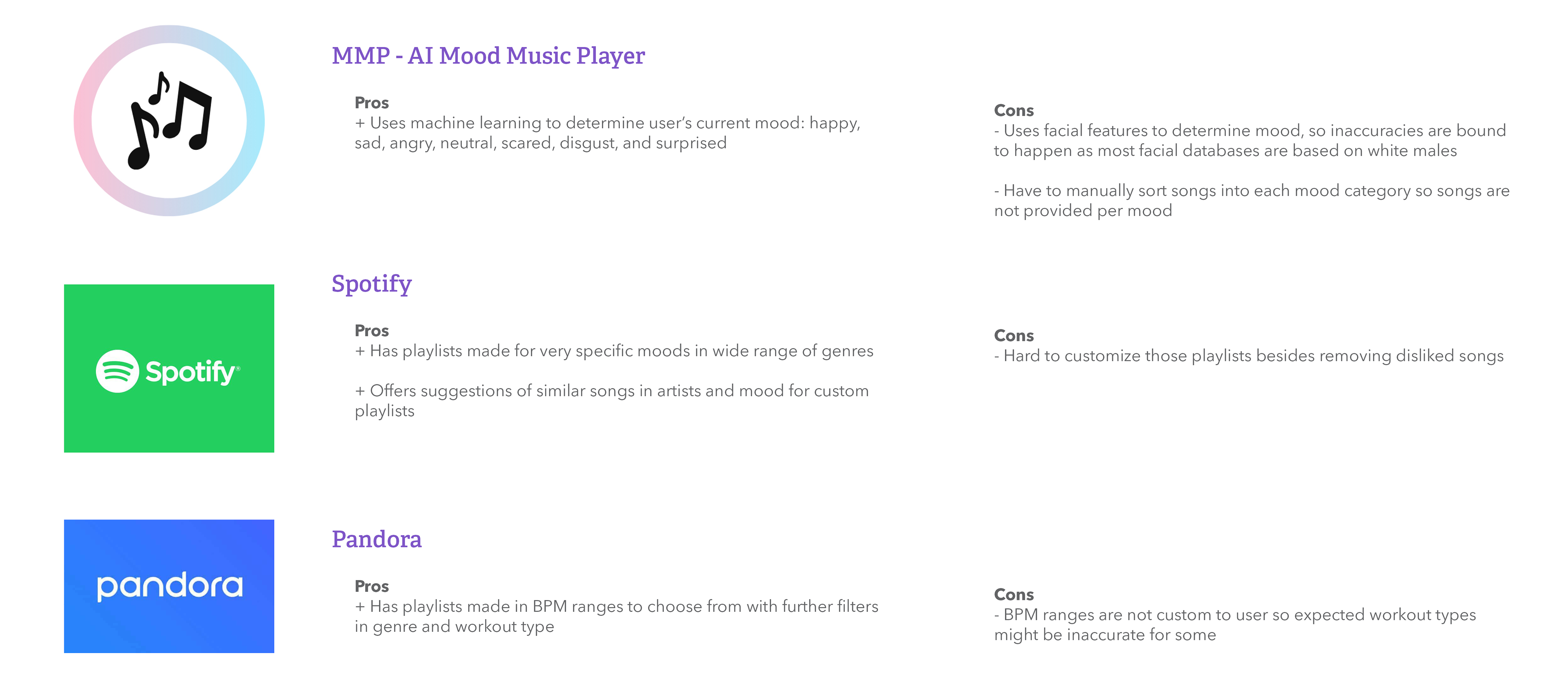
Interviews
I conducted interviews with several people that might be interested in the project and sent out a few open surveys.
Learning Goals
I wanted to gain insight into current solutions, complaints and motivations with music streaming.
It is important to understand who the users are, their lifestyle and determine what opportunities and needs exist for new music player apps, so that I can use these findings to shape the direction I take in developing a useful and intuitive product.
Key Findings
A majority of respondents claim to listen to music primarily for working out, focusing and relaxing.
Though spread fairly evenly, more than half of respondents are either very much into creating playlist or not at all.
For a majority of the time, almost 75% of respondents listen to their playlists in their entirety even if they do not like all the songs.
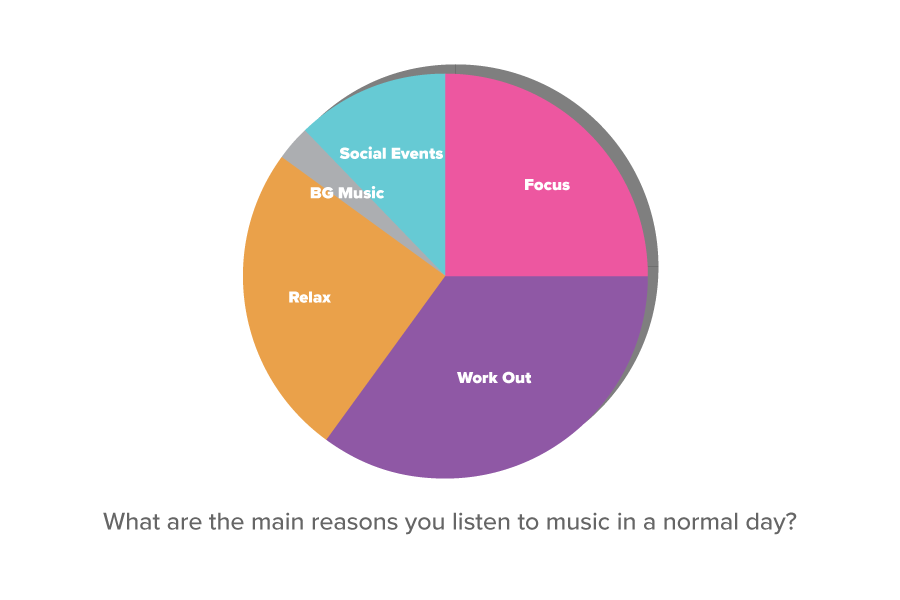
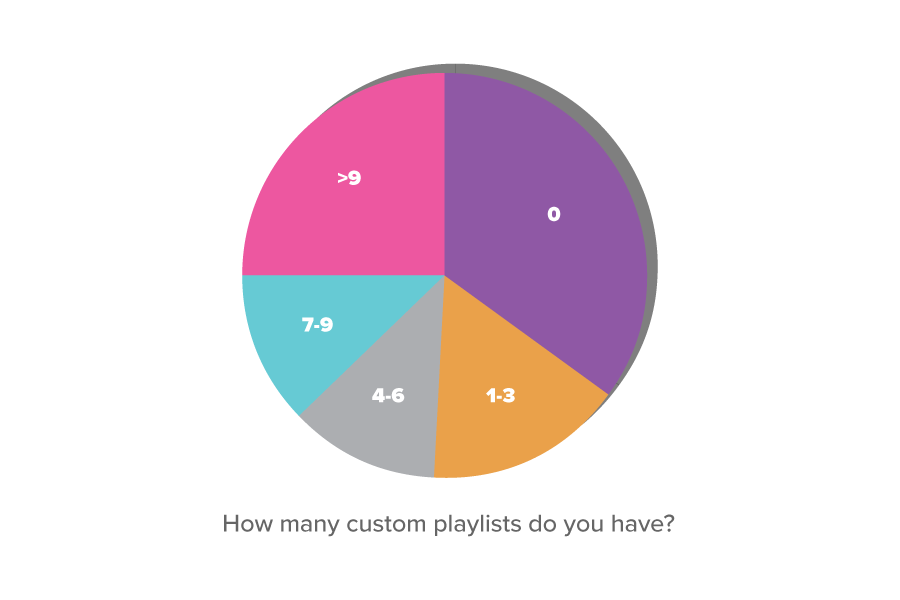
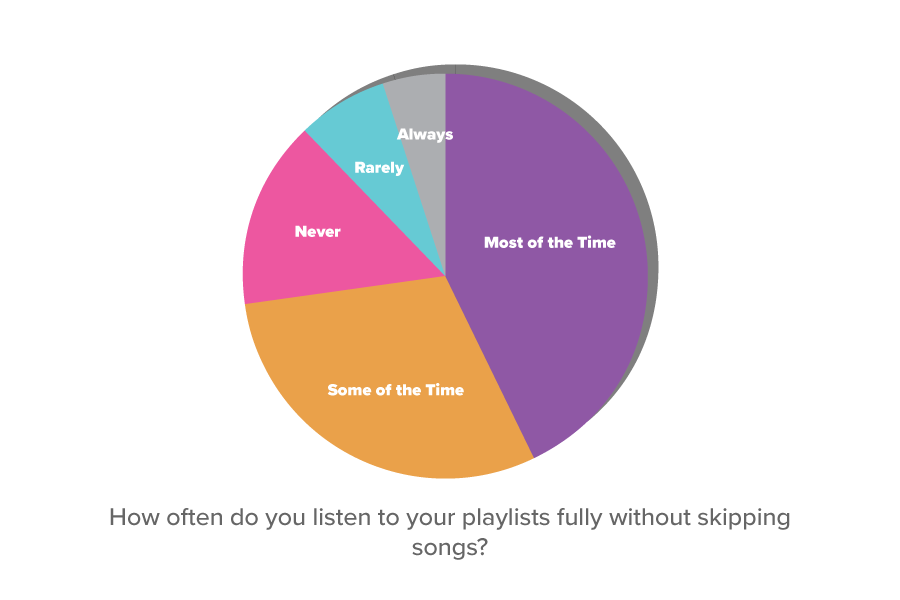
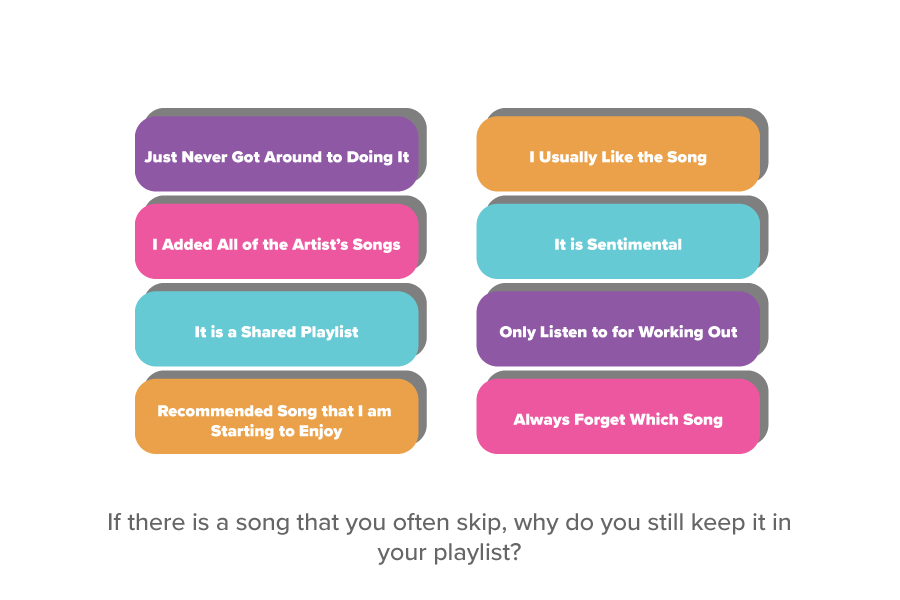
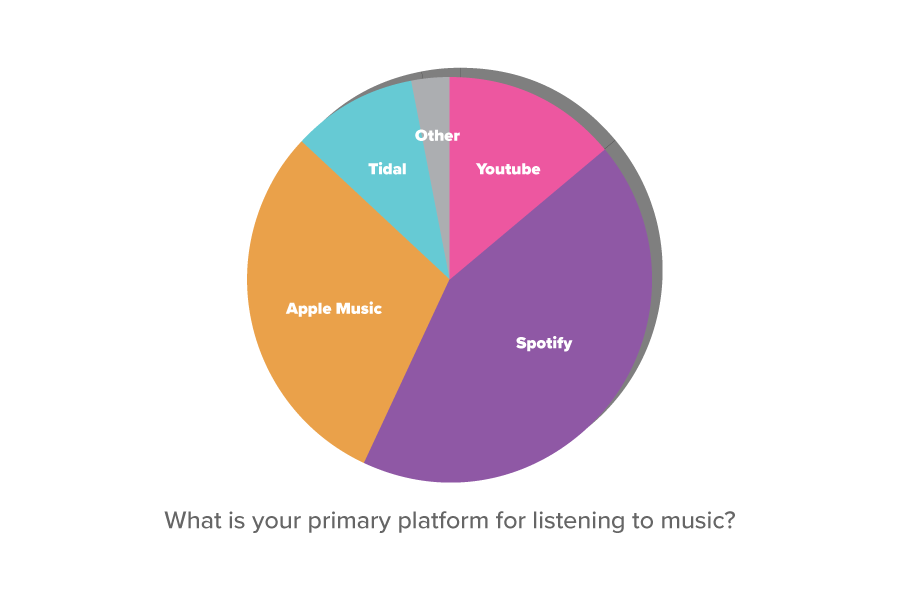
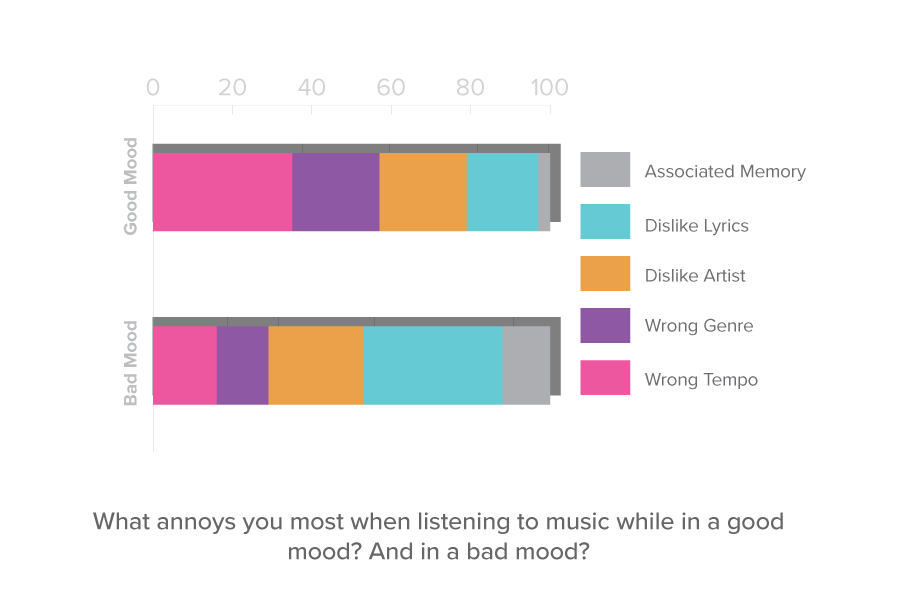
Personas
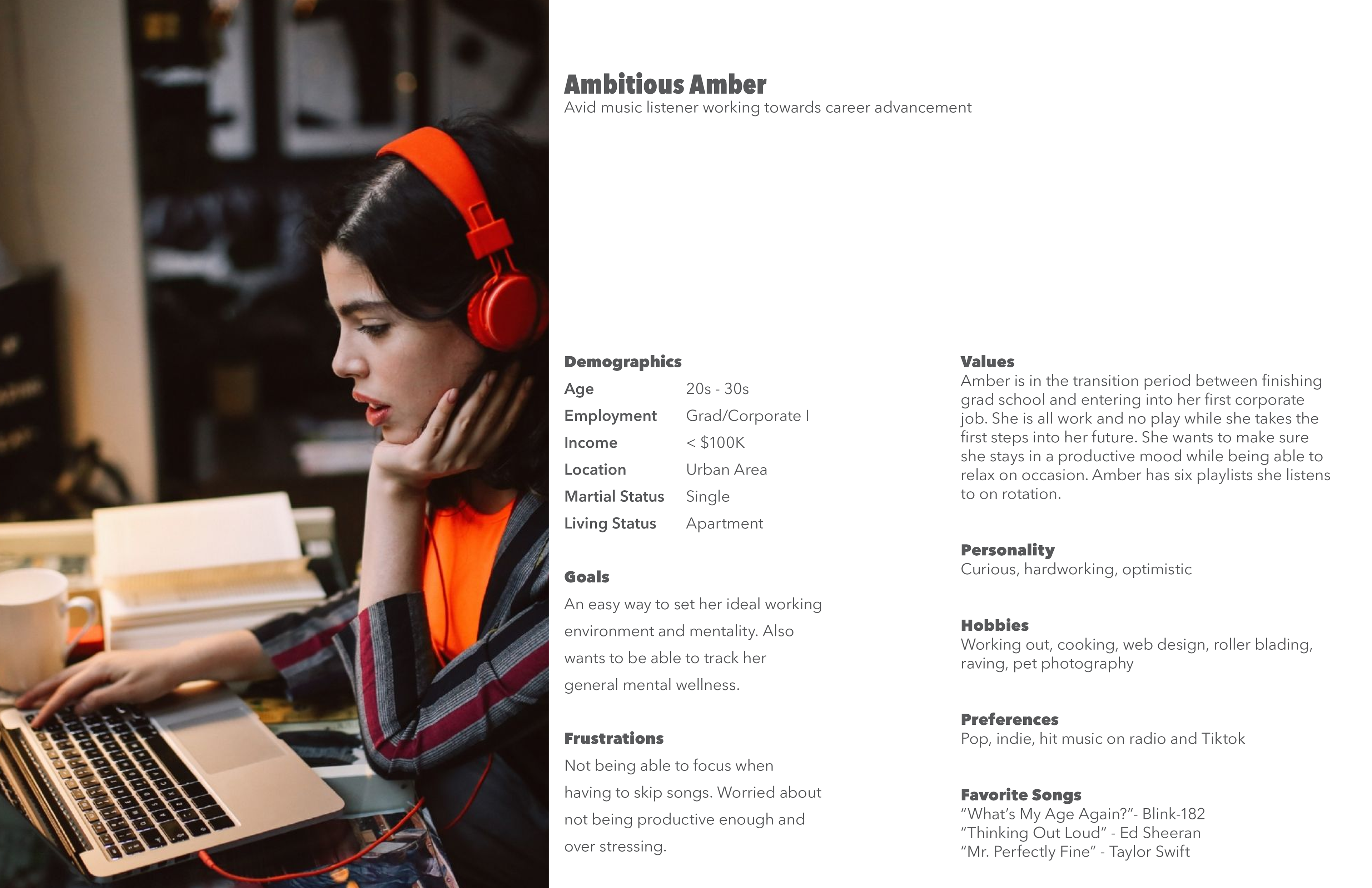
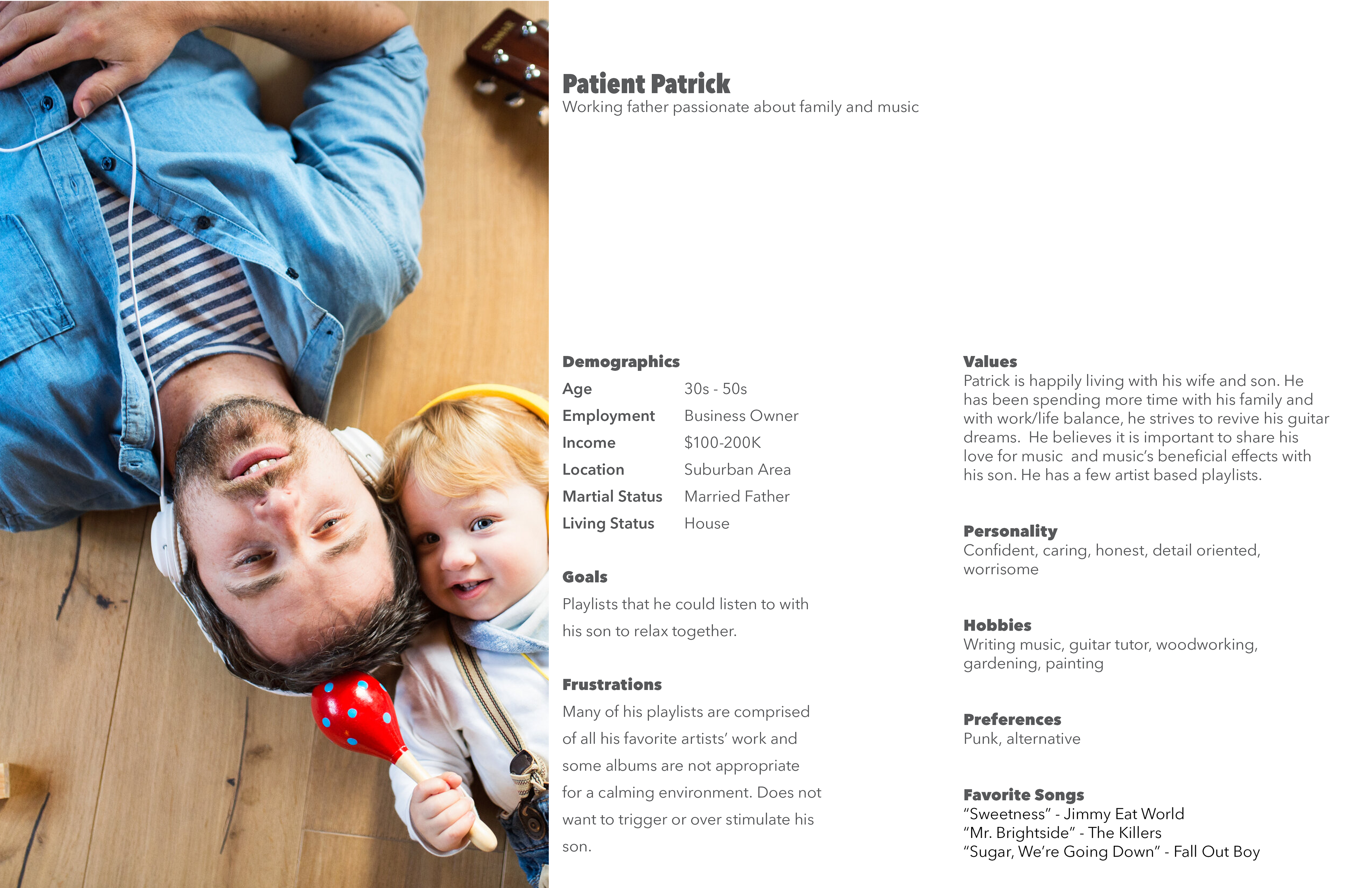
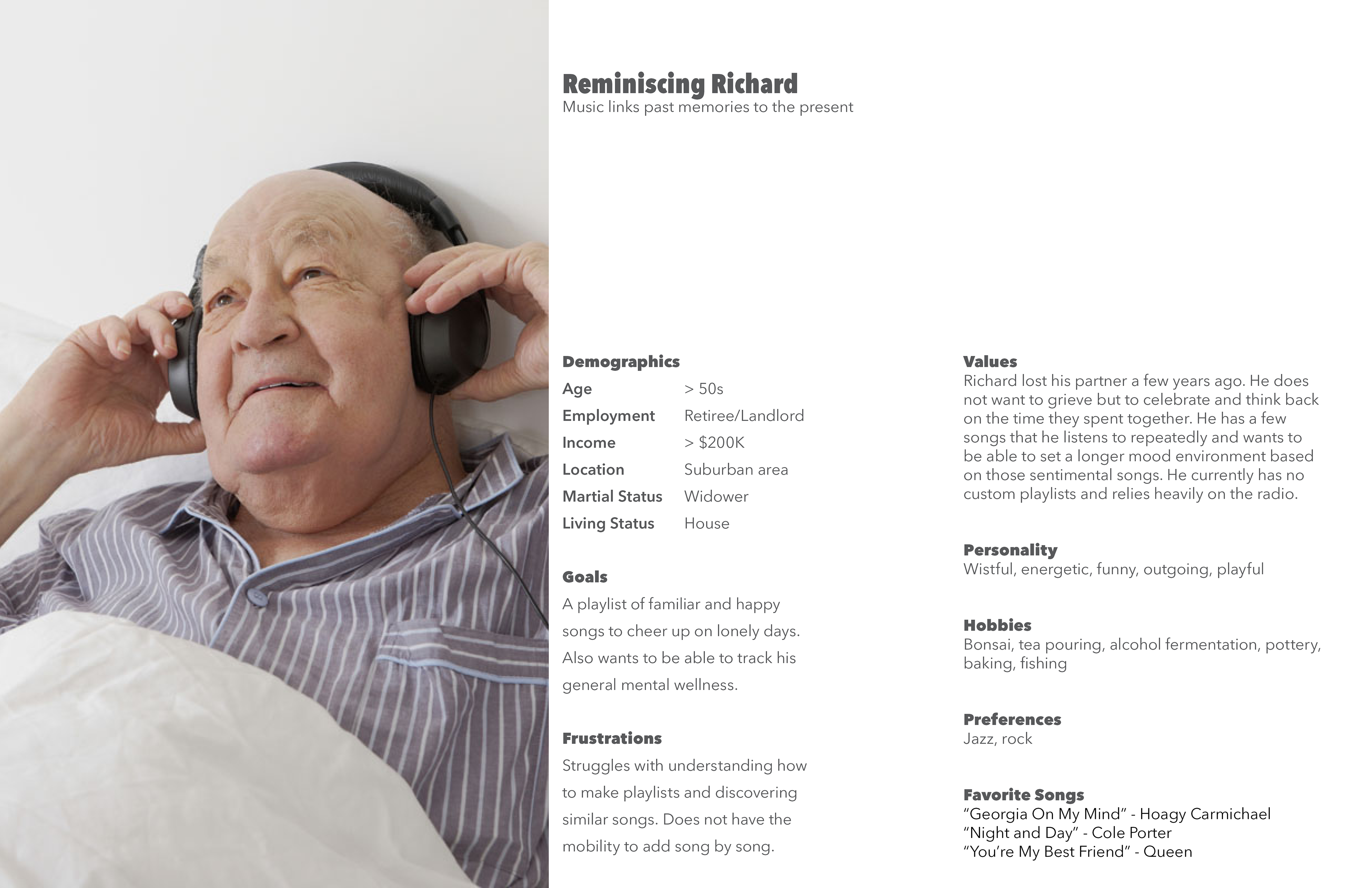
Design Process
Inspiration Board
Stylesheet
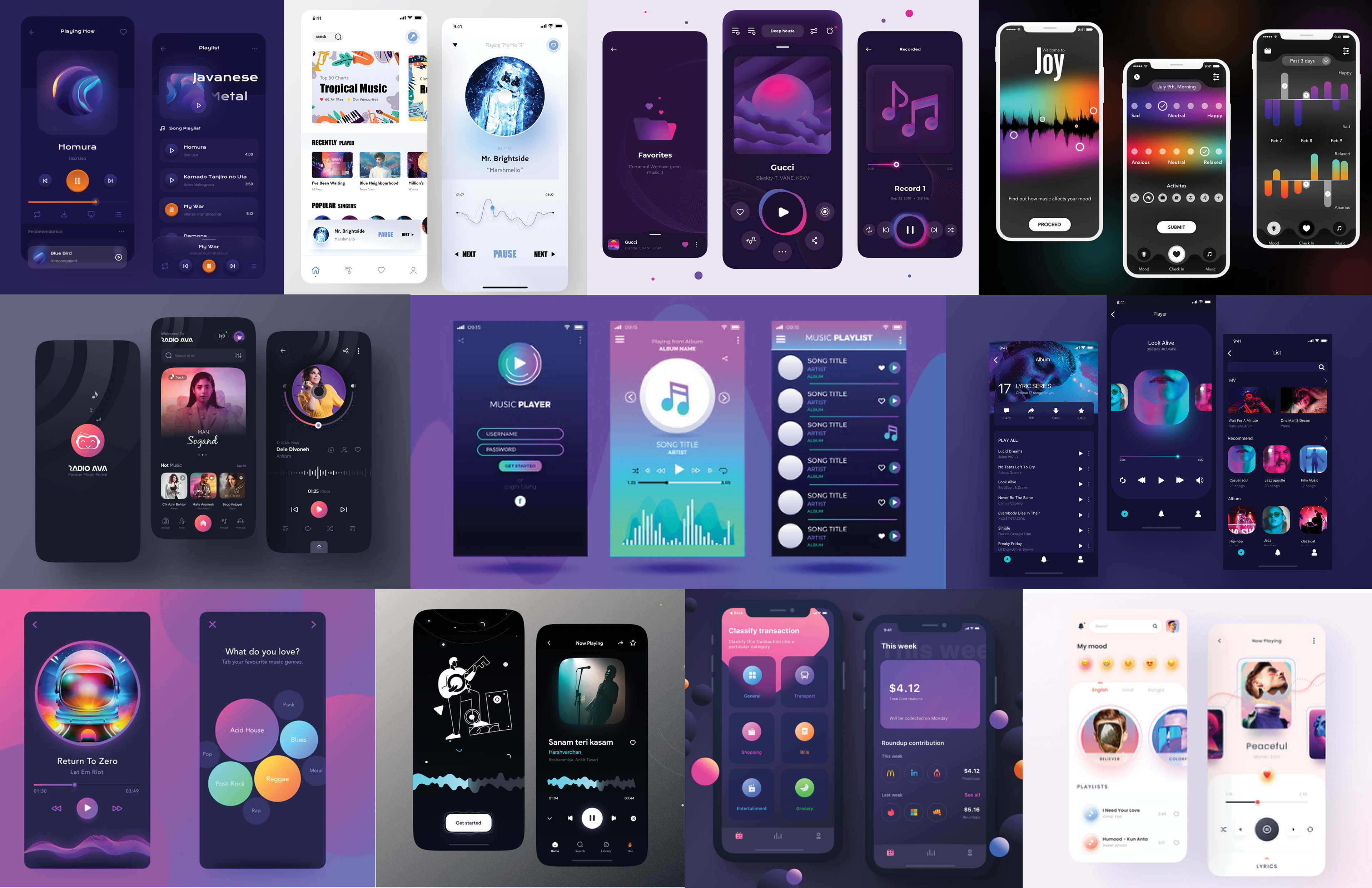
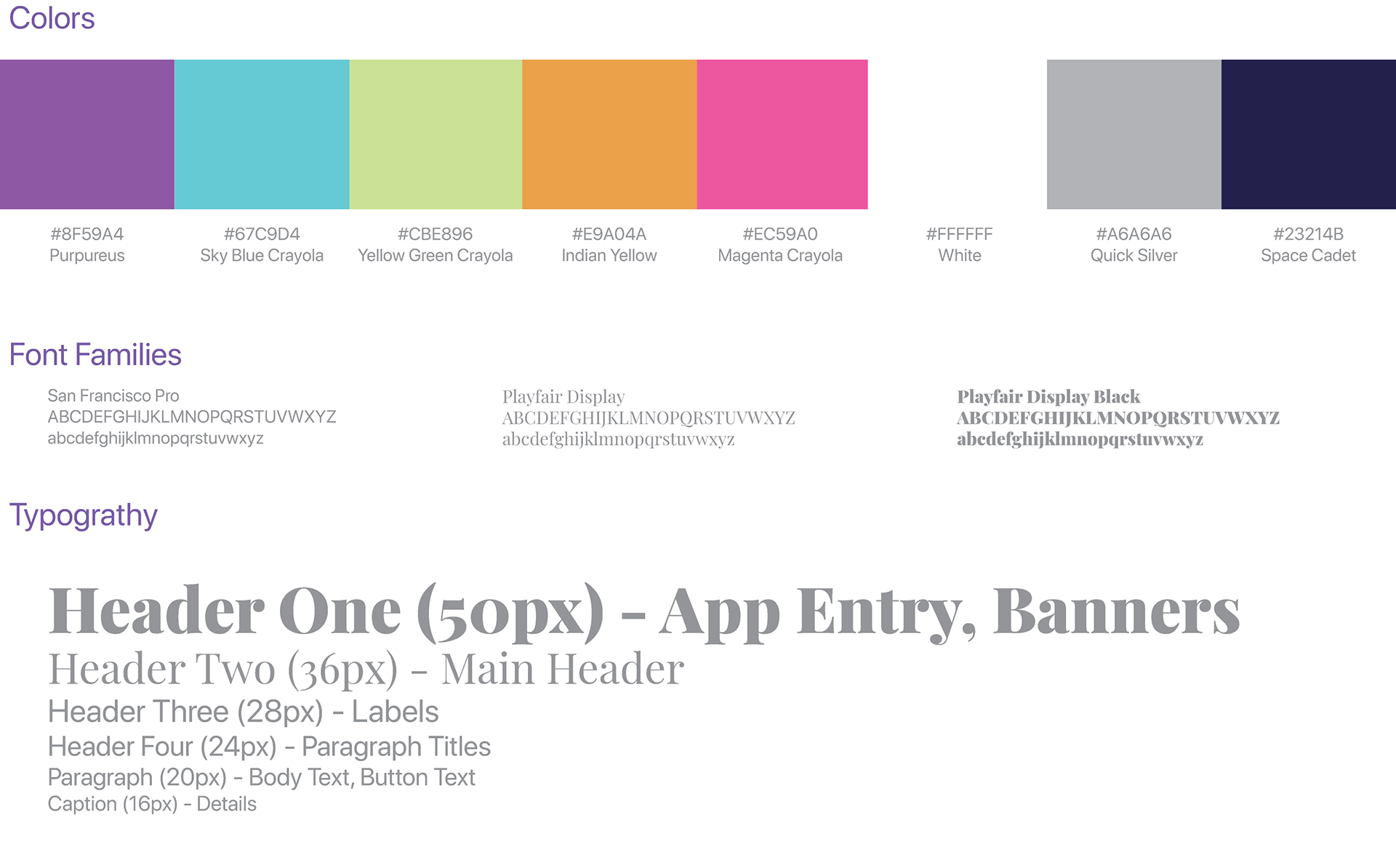
Logo
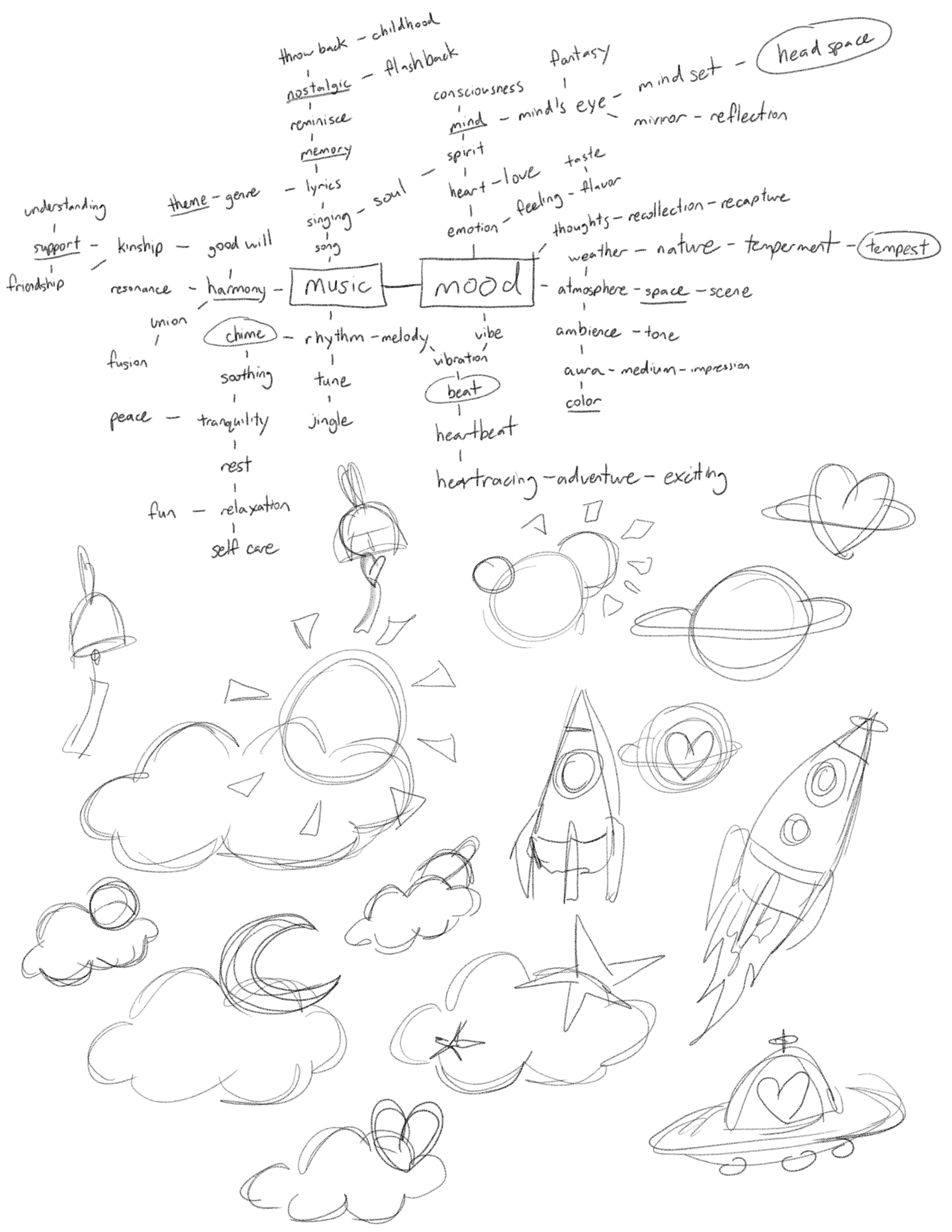
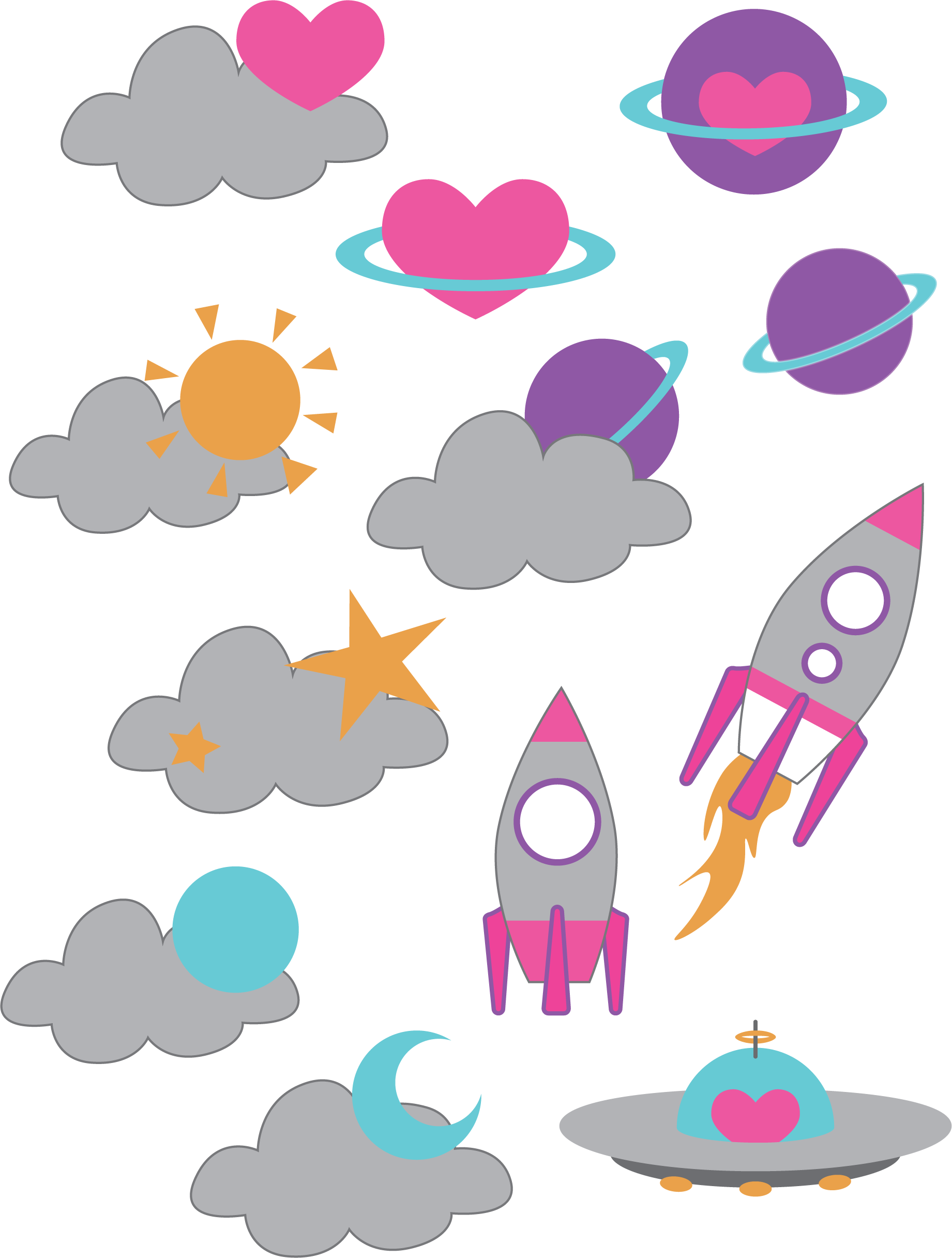
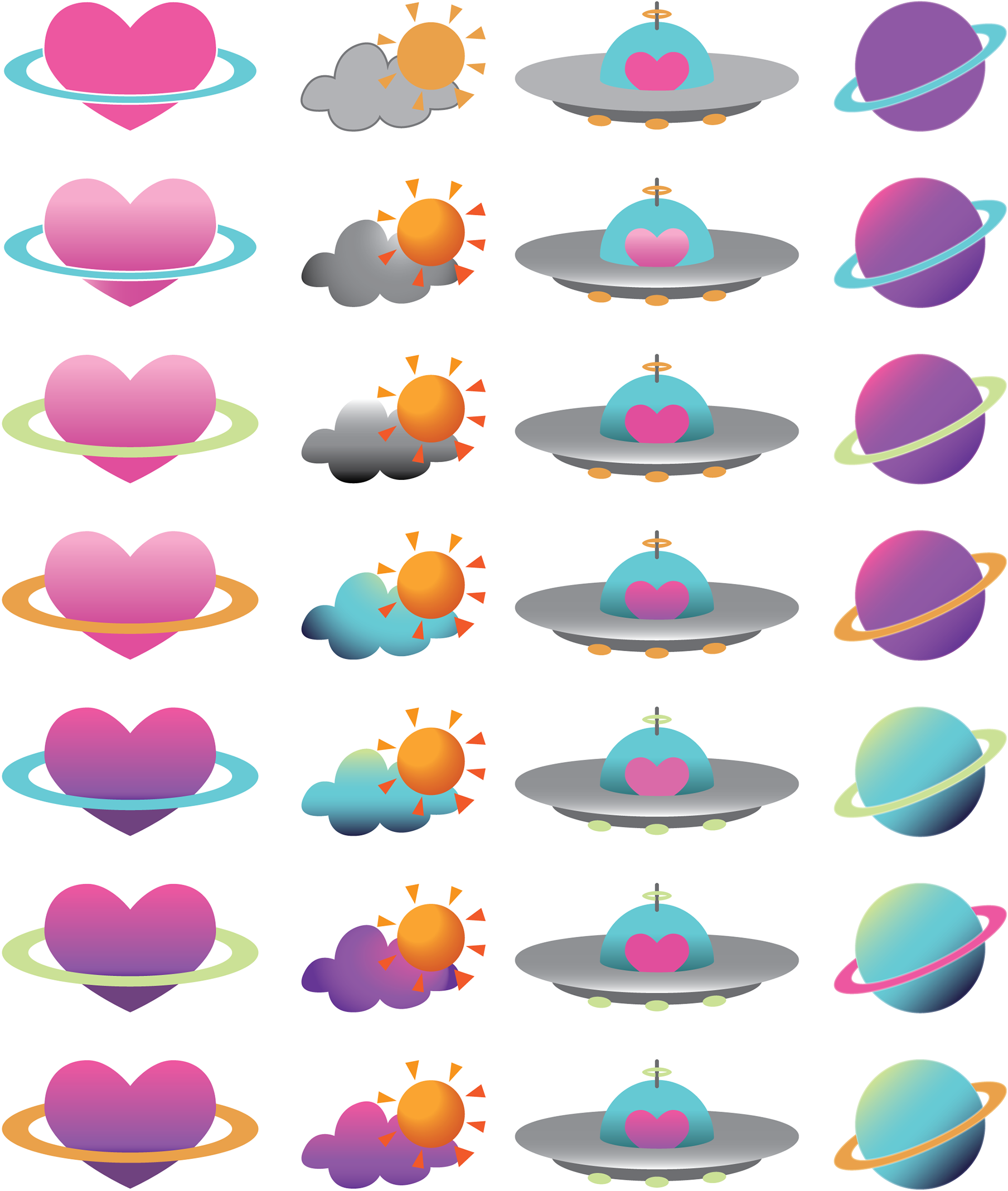
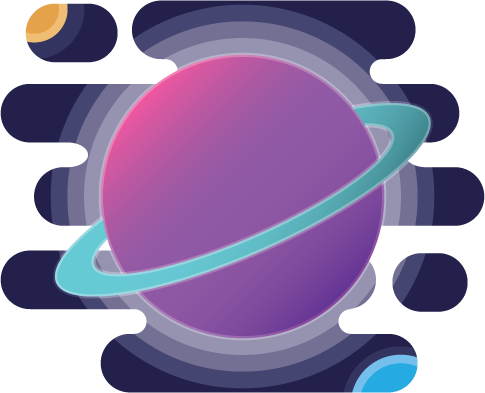
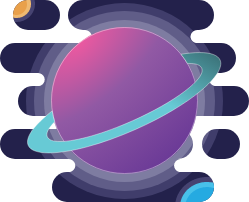
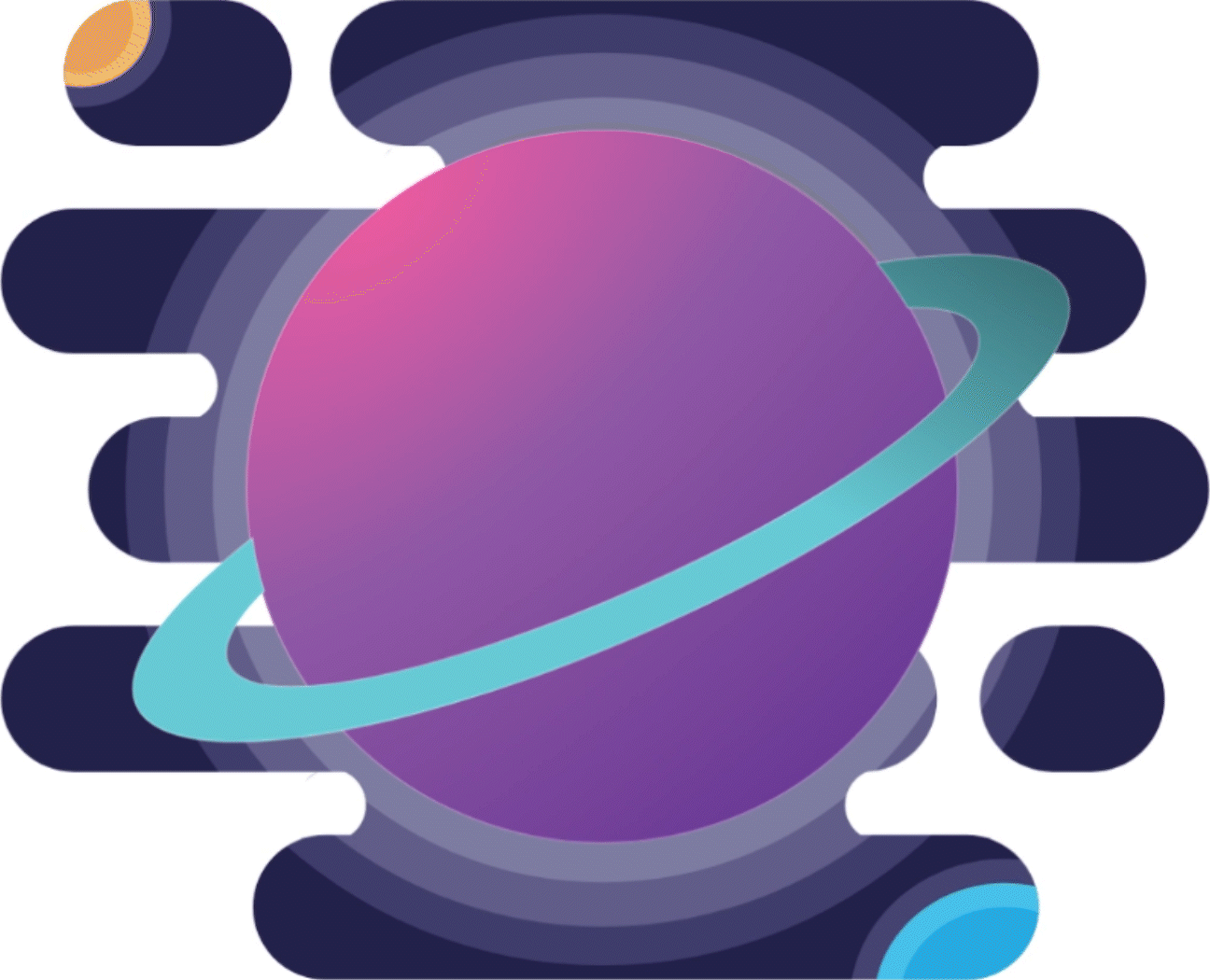
Initial Sketches
Creating a mindmap around the words "music" and "mood," I resonated with the concepts of headspace, tempest, and heartbeat. Further expanding on the theme of space, my sketches were predominantly inspired by the appearance of Jupiter's ring. Though heartbeats are the foundation of the app itself, the minimalist logo would allow the opportunity to also use the logo as an interactive component.
Creating a mindmap around the words "music" and "mood," I resonated with the concepts of headspace, tempest, and heartbeat. Further expanding on the theme of space, my sketches were predominantly inspired by the appearance of Jupiter's ring. Though heartbeats are the foundation of the app itself, the minimalist logo would allow the opportunity to also use the logo as an interactive component.
Illustrator vector logo vs Adobe XD recreation vs Adobe XD animation
Adobe XD's illustration functions are much more limited compared to Adobe Illustrator, but they are still great tools to mockup graphics and animations. Though I could have imported the vectors directly into the wireframe, it is important to rather recreate each layer, so the auto-animate function can recognize identically named components and their anchor points.
Adobe XD's illustration functions are much more limited compared to Adobe Illustrator, but they are still great tools to mockup graphics and animations. Though I could have imported the vectors directly into the wireframe, it is important to rather recreate each layer, so the auto-animate function can recognize identically named components and their anchor points.
Wireframes
Low Fidelity
The low-fidelity model allowed me to craft the foundation of the app. A large part of the wireframe is dedicated to set up: both the login process and uploading user data for determining current state. However, the rest of the pages are also essential parts of the app. They focus on choosing desired user mood, music selection, monitoring both BPM and HRV, and the user summary. Music selection would contain genre, artist and playlist search. Each song would have the option to add correlating moods for the app to improve future accuracy and preferences.
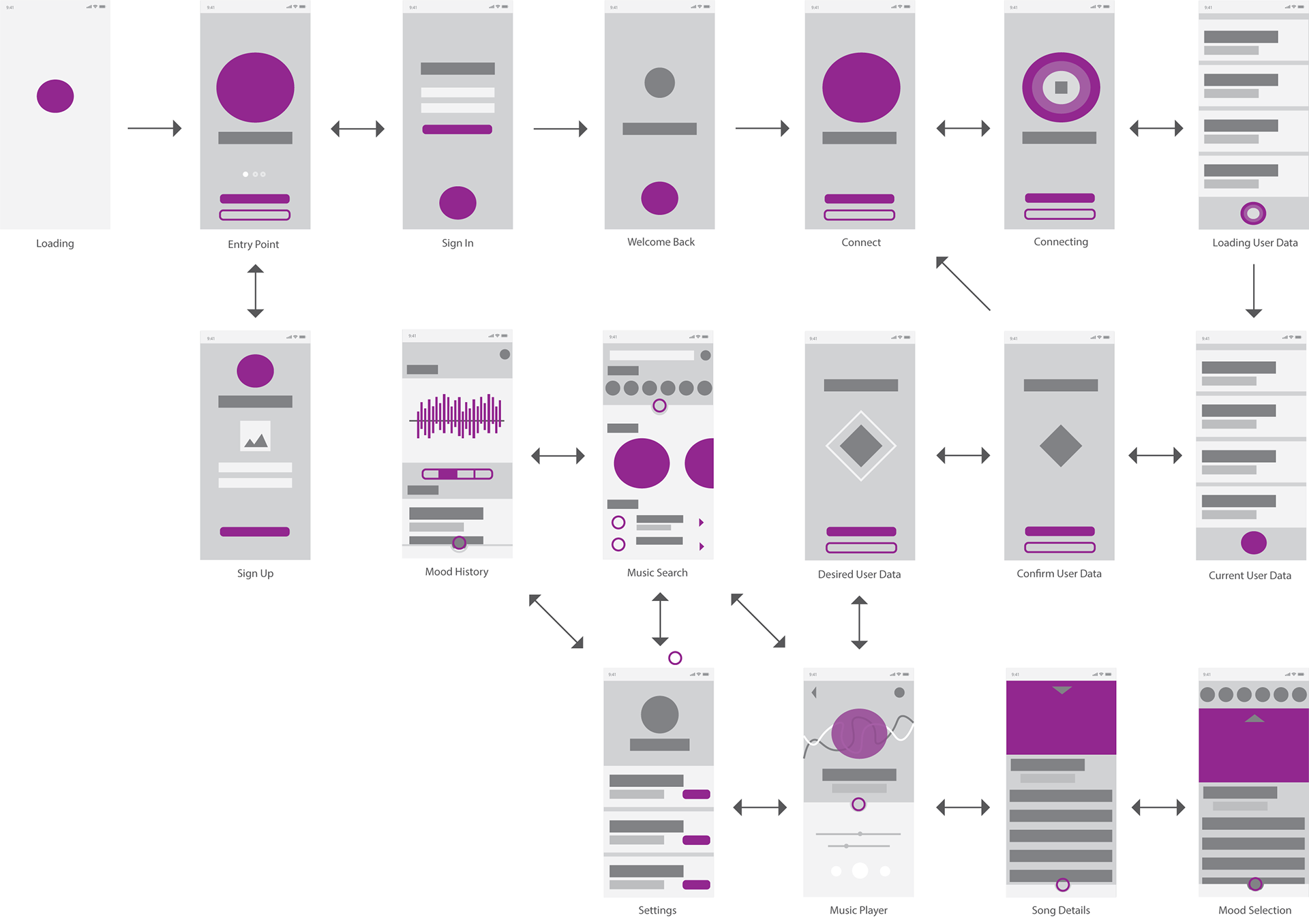
Medium Fidelity A/B
The medium-fidelity model hones into the details and actual composition. The style guide allows for a consistent look throughout the wireframe. More onboarding detail pages are added at this point along with rough drafts of graphics. The focus is now on the functionality of mood tracking and being able to look back on playlist history. The A/B differs in the login sequence, button placement and background. Usability tests show that users prefer B because the onboarding process makes more sense and the colors are easier on the eyes.
High Fidelity
The high-fidelity wireframes show all the pages which make up the app, including those that form the animations. The positives of both A and B middle fidelity wireframes were incorporated for the app to feel more user friendly.
Click here to see prototype
Core Pages
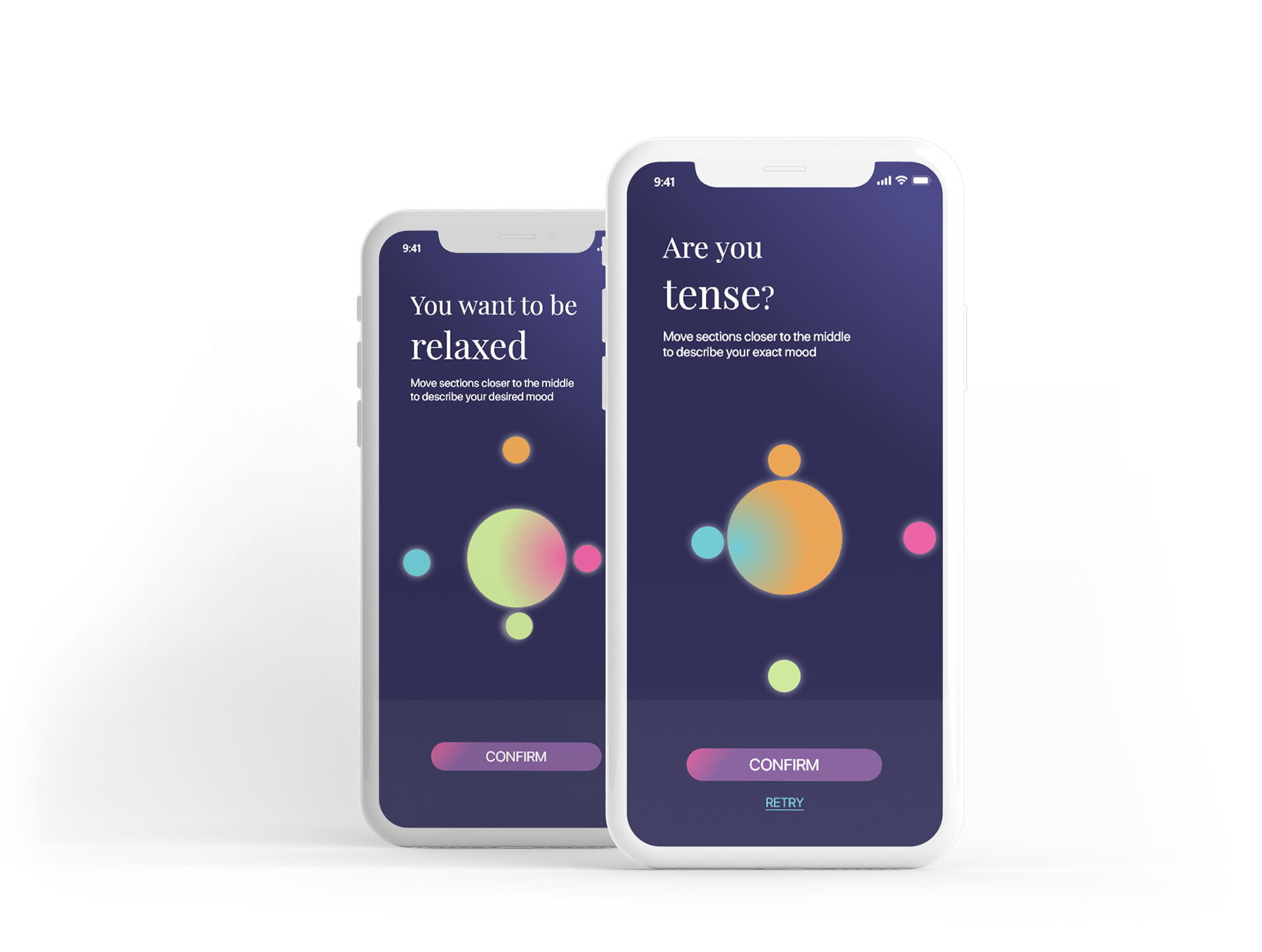
Mood Selection
The mood selection page imports the user's heart rate monitor data (BPM, resting heart rate, HRV & breaths pre minute) to estimate the correlating emotional valence and arousal. Using those two values, the app can determine where the user's current mood lies on the emotion cycle.
From there, the user will set the listening session's goal mood by moving the four emotion markers (positive/negative valence & positive/negative arousal). The closer to the center planet, the higher the value. Users will be shown one of 17 mood ranges as the markers are moved.
Suggested Mood Playlist
The suggested mood playlist page offers a recommended listening process from songs that match the user's current mood to songs that match the user's desired mood. Songs gradually transition to lower or raise BPM. With machine learning, the app can learn song preferences to also affect HRV. This page allows users to do a quick view of the playlist and do an initial filter if necessary.
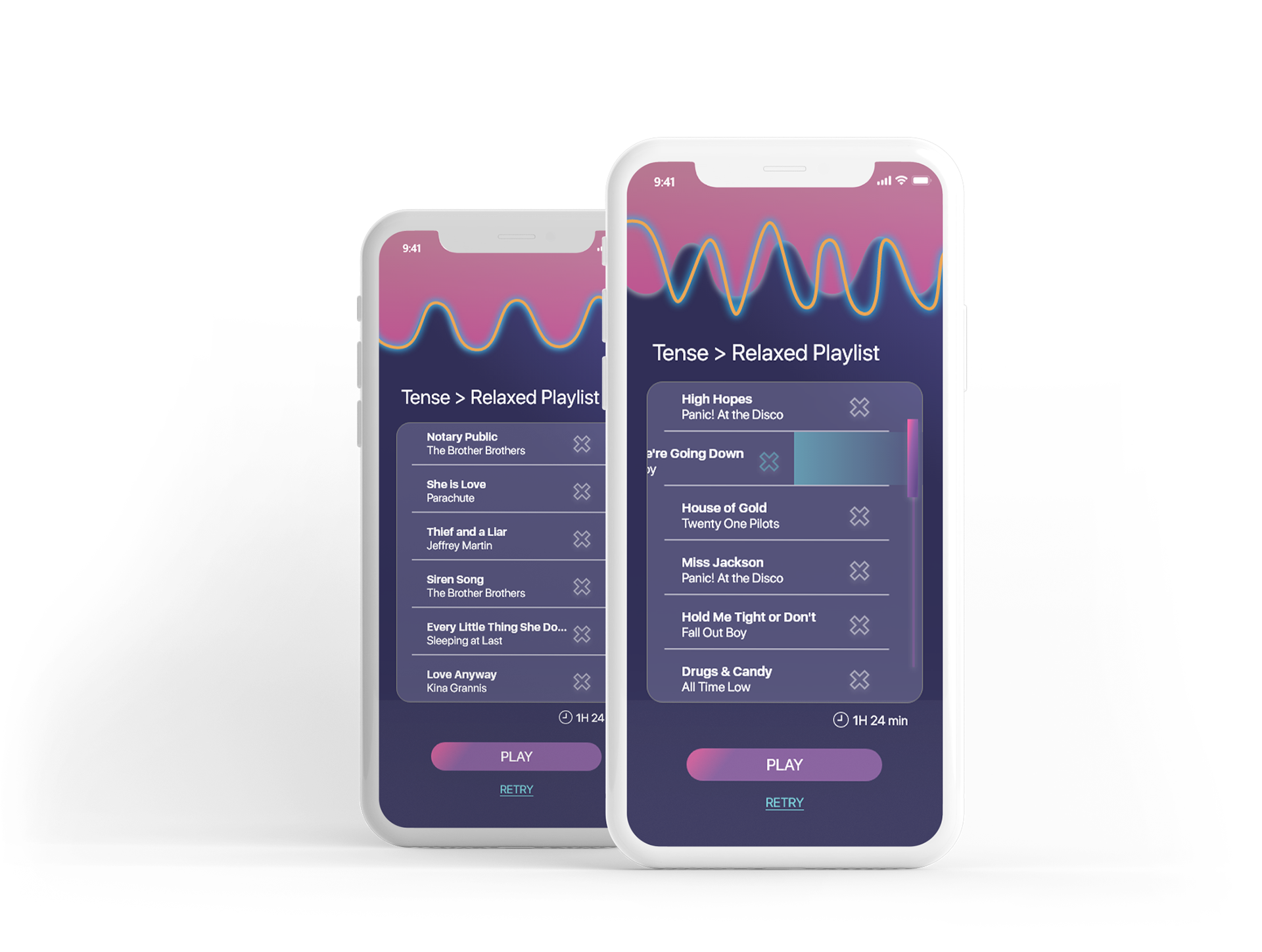
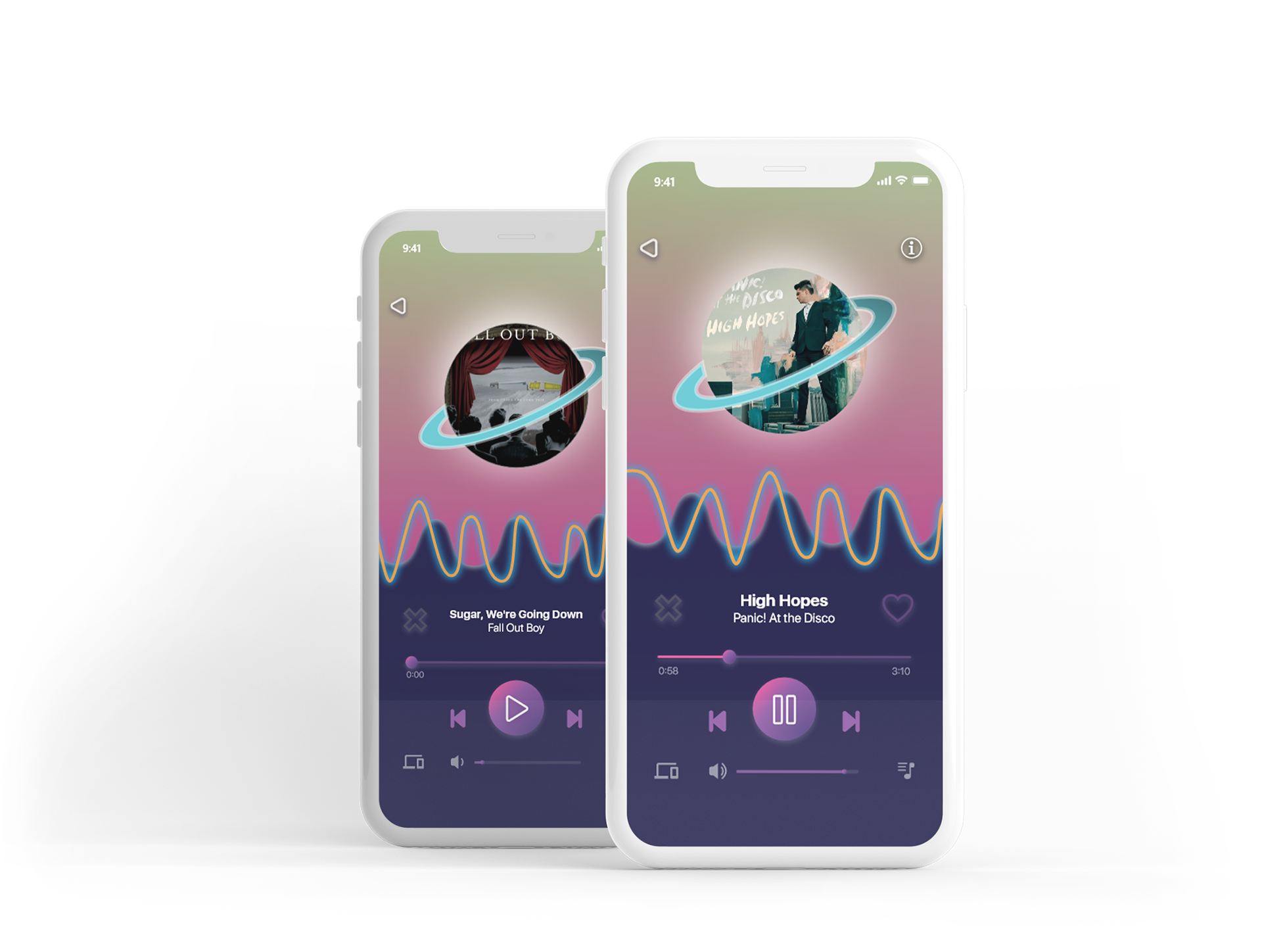
Music Player
The music player is the main page users will see during their session. It shows the current song being played, and their mood transition process. This is shown visually through two lines: one for current and one for goal. To better demonstrate the hierarchy, the goal heartbeat (combination of BPM and HRV) line is shown as the static backdrop in the color(s) of the desired mood. The active line is in the current mood color(s) and will show the progress determined by the user's heart rate monitor. Once both lines are parallel, the listening session will end. Users can also favorite or remove songs. Favorited songs will be included in all similar future playlists while removed songs will never be played again for similar moods.
Explore
The explore page gives users the opportunity to search for and manually enter certain songs into emotion categories. This allows the app to better learn user preferences and will improve future suggested playlists. The better the app understands a user's emotional links to songs, the better it will understand which songs would accurately affect both BPM and HRV. The explore page will more importantly show recent mood playlists and mood history. Users can listen to their past sessions if they wish to, or they can look at their mood trends to track their own mental wellbeing. While on the explore page, users can still view their active heartbeat line and their goal heartbeat line.
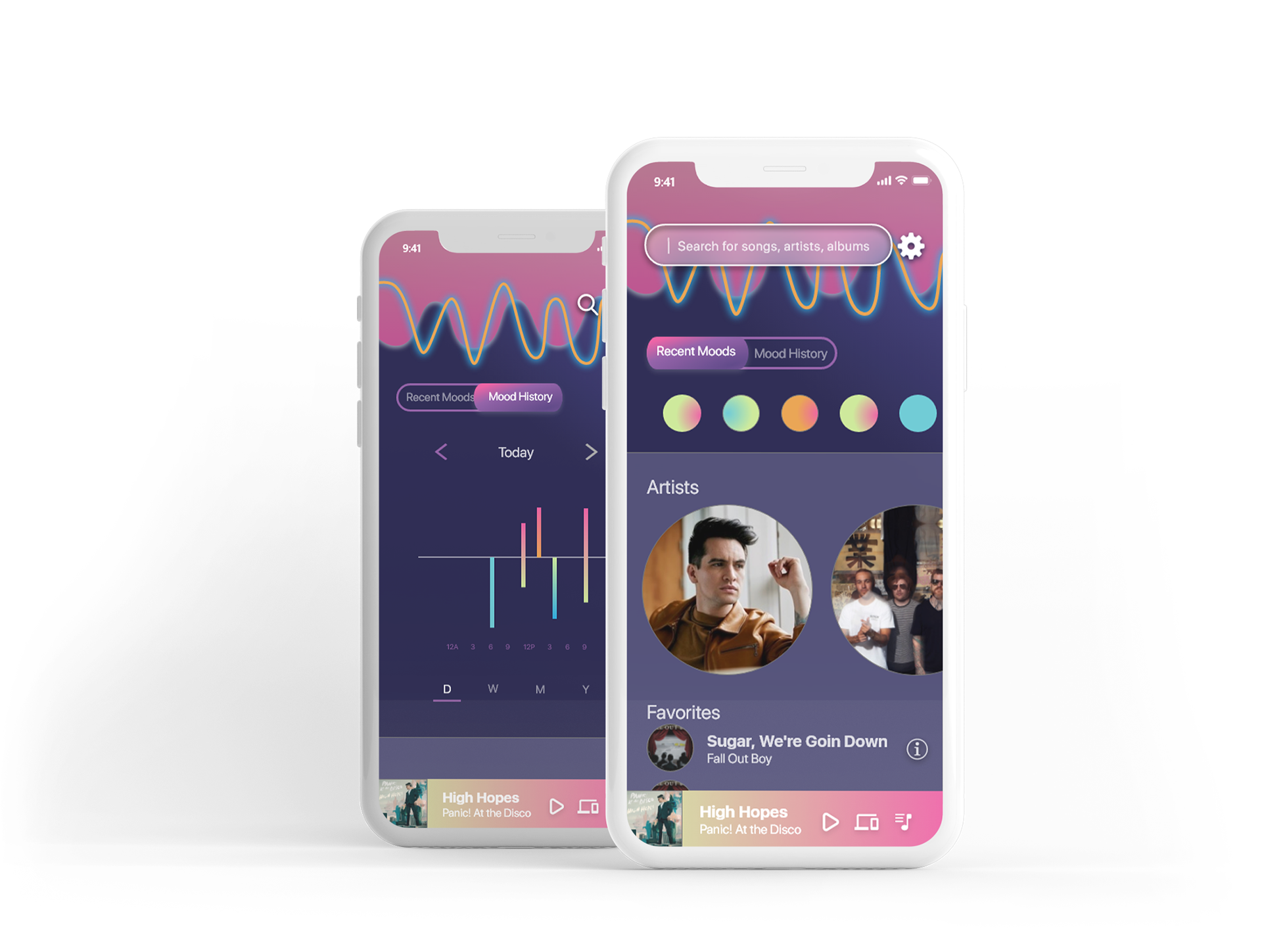
Vivifying the App
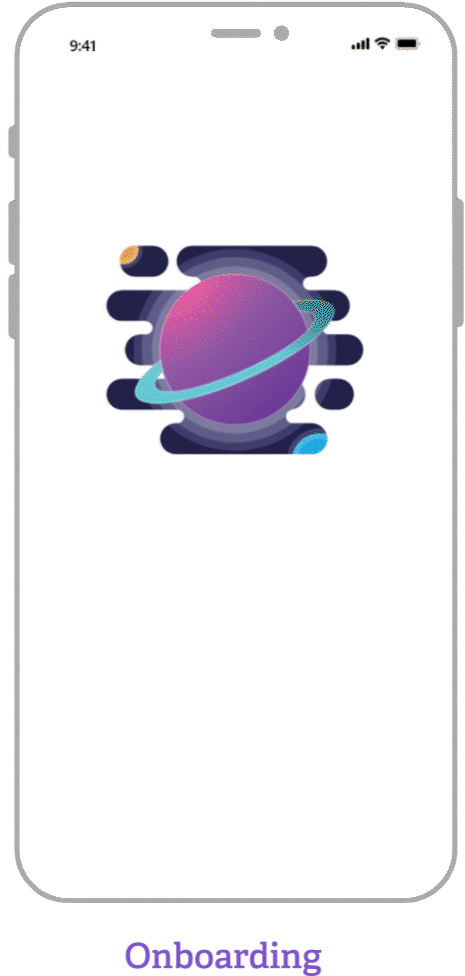
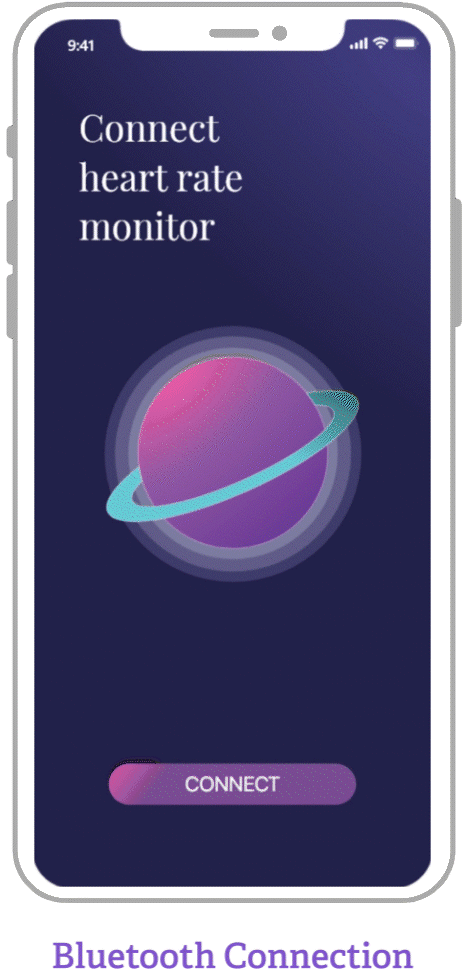
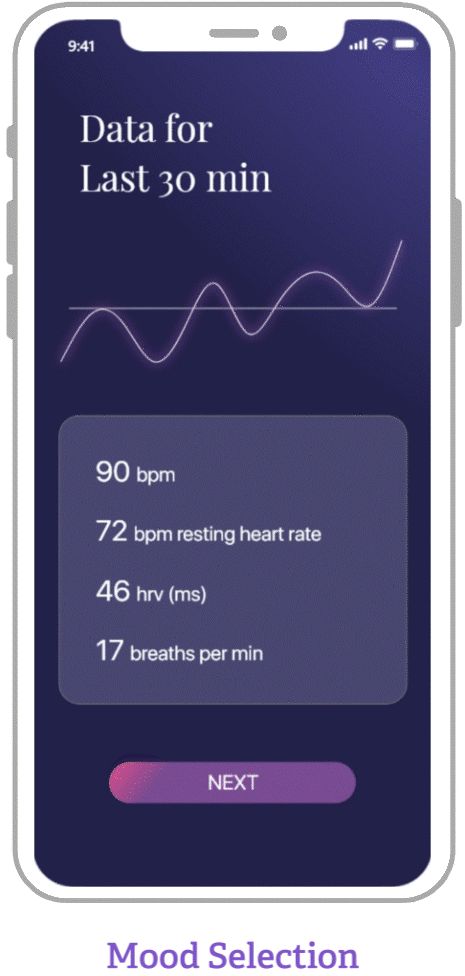
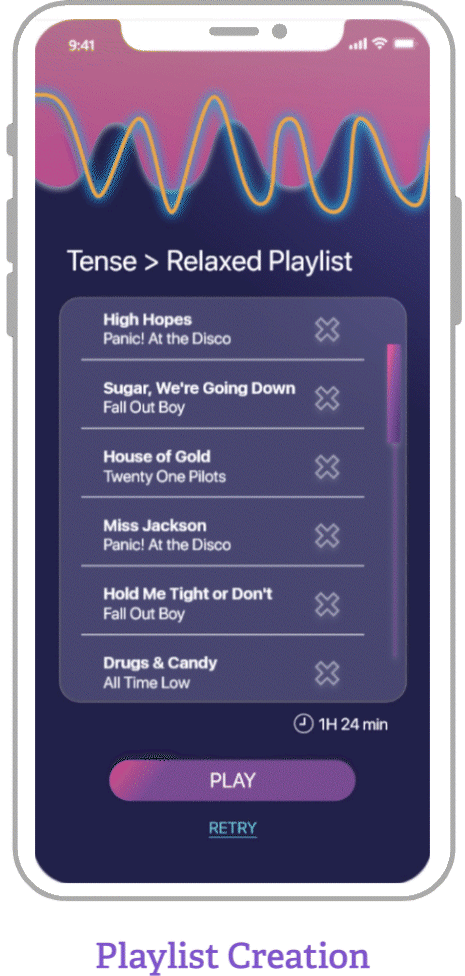

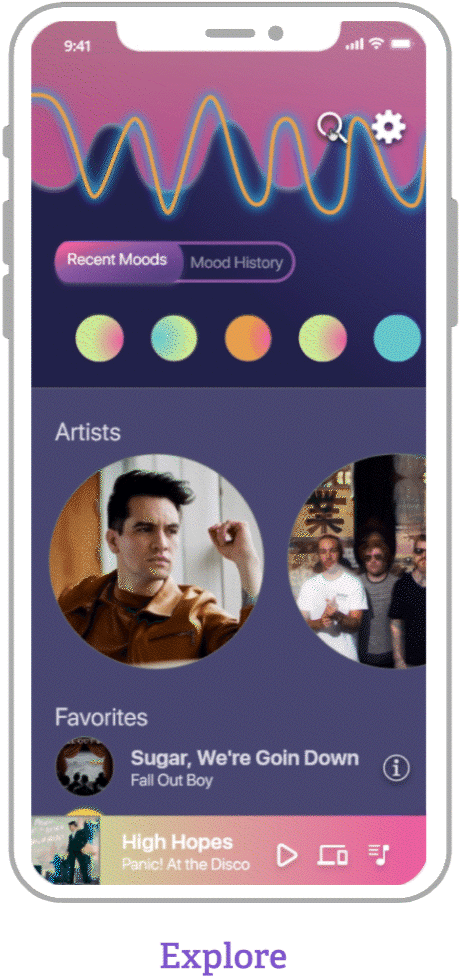
Next Steps
As my first UI/UX project taken from concept to full fidelity, I learned a lot about the capabilities of Adobe XD. I want to further explore the animation capabilities especially in the process of creating button components. They add much more interactivity while reducing the number of pages needed for animations. Components are truly my favorite part of Adobe XD in its ability to bring prototypes to life.
I will continue to build out the features for intuitive use and more accurate song selections . At the moment, the app only focuses on the HRV of the user, but I want to do more research into the correlation between respiration rate and skin temperature (both features are already possible in Fitbits). It would make the emotion analyses more accurate per user by combining manual inputs with heartrate monitor data. I will also consider the ability for the user to choose the simplicity of the interface to provide a more desirable app for a broader range of users. Once that is done, I will conduct another round of usability testing before iterating the website version of the app to widen the scope of functionality.
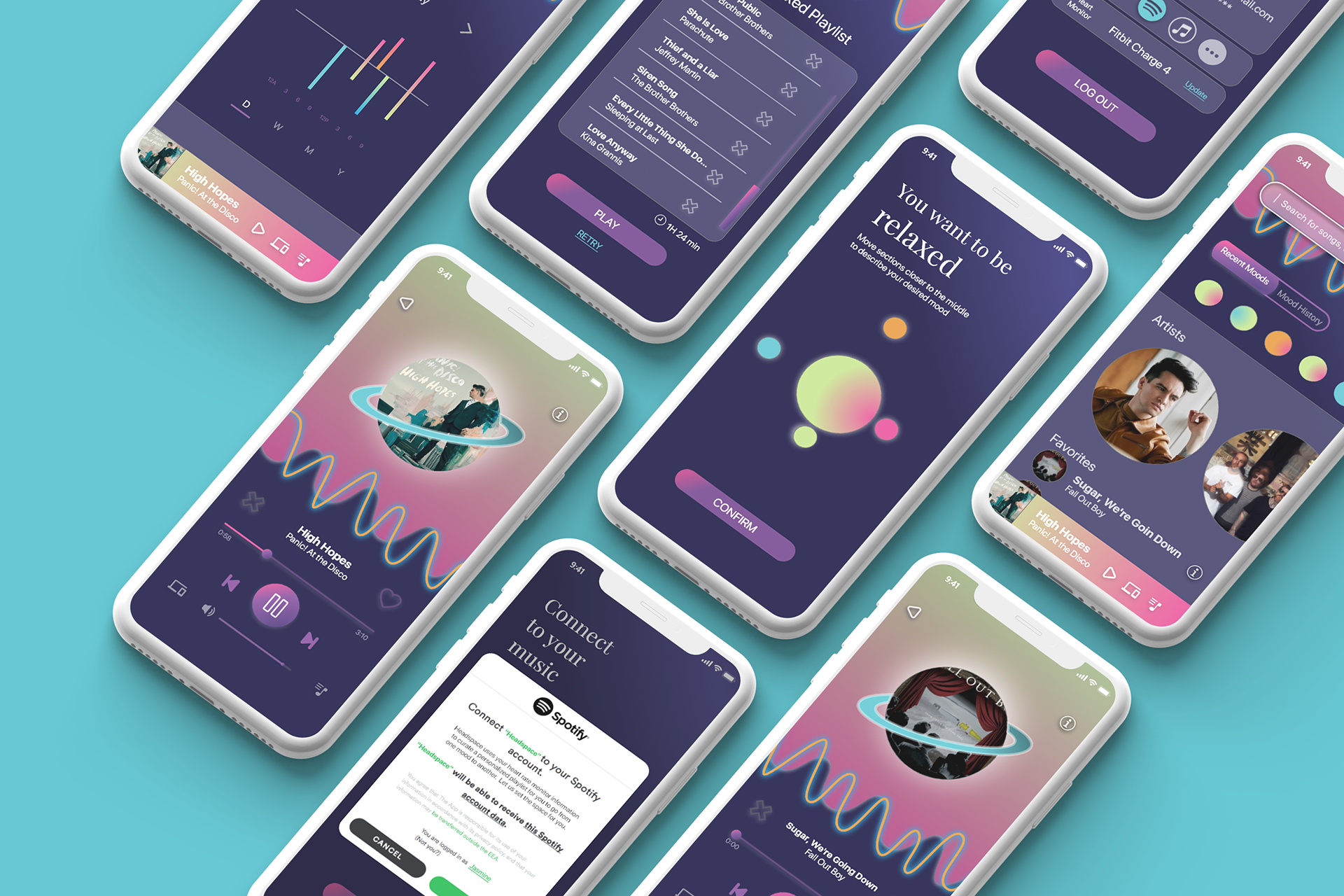
References
Choi, Kwang-Ho et al. “Is heart rate variability (HRV) an adequate tool for evaluating human emotions? - A focus on the use of the International Affective Picture System (IAPS).” Psychiatry research vol. 251 (2017): 192-196. doi:10.1016/j.psychres.2017.02.025
Dzedzickis, Andrius; Kaklauskas, Artūras; Bucinskas, Vytautas. 2020. “Human Emotion Recognition: Review of Sensors and Methods” Sensors 20, no. 3: 592. https://doi.org/10.3390/s20030592
Gupta A, Ahmed B. “Experience of listening to music on patient anxiety during minor oral surgery procedures: a pilot study.” British Dental Journal. 2020 Jan;228(2):89-92. DOI: 10.1038/s41415-019-1162-1.
Hernandez-Ruiz, Eugenia, et al. “What Makes Music Relaxing? An Investigation into Musical Elements.” Psychology of Music, vol. 48, no. 3, May 2020, pp. 327–343, doi:10.1177/0305735618798027.
Miller JS, Terbizan DJ. “Clinical Outcomes of Different Tempos of Music During Exercise in Cardiac Rehabilitation Patients.” Int J Exerc Sci. 2017 Sep 1;10(5):681-689. PMID: 28966708; PMCID: PMC5609668.
Pereira, Carlos Silva et al. “Music and emotions in the brain: familiarity matters.” PloS one vol. 6,11 (2011): e27241. doi:10.1371/journal.pone.0027241
Schubert, Emery. “The Influence of Emotion, Locus of Emotion and Familiarity upon Preference in Music.” Psychology of Music, vol. 35, no. 3, July 2007, pp. 499–515, doi:10.1177/0305735607072657.
van den Bosch, Iris et al. “Familiarity mediates the relationship between emotional arousal and pleasure during music listening.” Frontiers in human neuroscience vol. 7 534. 5 Sep. 2013, doi:10.3389/fnhum.2013.00534
van Dyck, Edith, et al. “Adopting a Music-to-Heart Rate Alignment Strategy to Measure the Impact of Music and Its Tempo on Human Heart Rate.” Musicae Scientiae, vol. 21, no. 4, Dec. 2017, pp. 390–404, doi:10.1177/1029864917700706.
Wu, Yan et al. “How Do Amusement, Anger and Fear Influence Heart Rate and Heart Rate Variability?.” Frontiers in neuroscience vol. 13 1131. 18 Oct. 2019, doi:10.3389/fnins.2019.01131
Zhu J, Ji L, Liu C. “Heart rate variability monitoring for emotion and disorders of emotion.” Physiol Meas. 2019 Jul 1;40(6):064004. doi: 10.1088/1361-6579/ab1887. PMID: 30974428.